The challenge of Analysis Ready Data in Earth Observation
Towards seamless integration: The quest for interoperable and harmonized EO data
Towards seamless integration: The quest for interoperable and harmonized EO data
One of the major challenges when building applications using EO data is having “clean-ready-to-use-data”, or what we most commonly refer to as Analysis Ready Data (ARD); that is, data that we can use almost out-of-the-box and do time-series analysis, train machine learning models and derive high-level products without spending huge amounts of time in data preparation in order to work on the actual task.
NOTE 1: Although there are efforts towards non-optical ARD (e.g. Sentinel-1 ARD¹), this post covers optical ARD.
NOTE 2: I have split this article into two sections.
In the first section I explore what we “traditionally” consider as ARD and ARD products such as the Landsat ARD and the Harmonized Landsat and Sentinel-2 (HLS) ARD. I follow ARD-related workshops and highlight several points I find interesting in the presentations. I also dedicate a section related to Planetscope data and Planet’s efforts towards creating an ARD product.
In the second section, I review a recent study conducted by the Landsat Advisory Group exploring earth observation products, including data, algorithms, and workflows. I dedicate Section 2 of this article on it, as I consider it an “expanded” version of ARD.
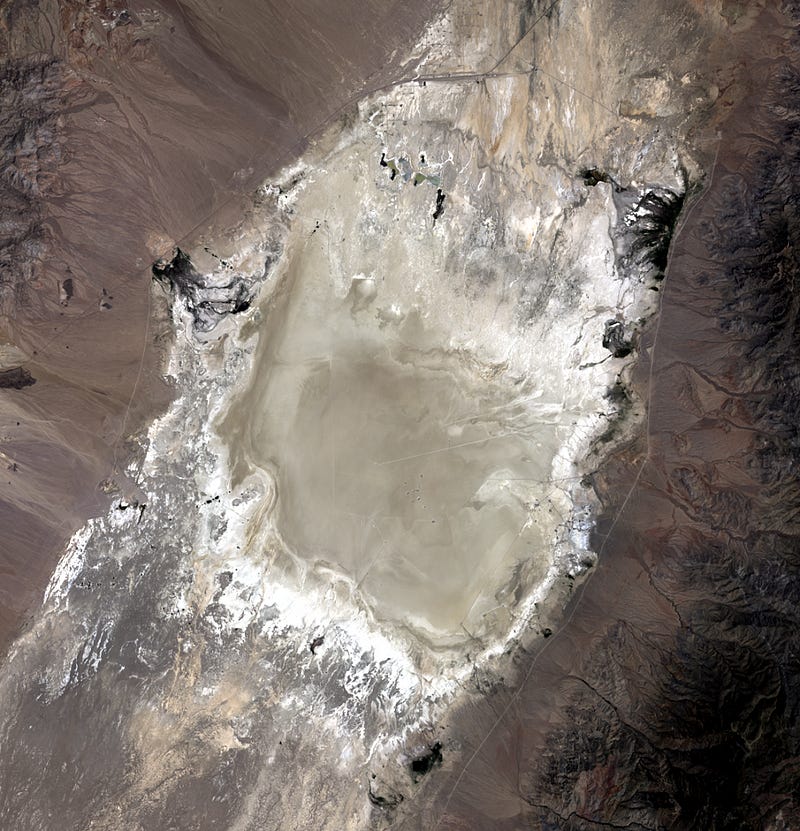
Section 1
Defining ARD
There are several definitions of ARD:
“Data that have been processed to allow analysis with a minimum of additional user effort are often referred to as Analysis Ready Data (ARD).”²
“(CEOS) Analysis Ready Data (CEOS-ARD) are satellite data that have been processed to a minimum set of requirements and organized into a form that allows immediate analysis with a minimum of additional user effort and interoperability both through time and with other datasets.”³
products that enable the scientific community and non-expert data users to spend more time conducting research into urgent issues in earth system science, such as climate change, food-energy-water nexus, and other priorities, rather than data cleaning and standardisation procedures³
The last one is not a definitation but rather the goal of ARD products. However, there is something common in all of them; the need for reducing usage complexity.
Why we want (“traditional”) ARD?
Interoperability and Data Quality
Ideally, users would be able to examine any pixel over time seamlessly, in a “stackable” data cube.
ARD provides consistent formats, projections, and coordinate systems across different satellite datasets, enabling seamless integration and analysis.
More than that, ARD is usually accompanied with QA assets; quality assessment information about the data (QA bands for cloud, haze, snow, flagged pixels due to poor quality, etc).
Data compatibility for time-series analysis
ARD facilitates time-series analysis by providing consistent and compatible data across different satellite sensors and time periods. It enables monitoring and comparison of environmental changes, land cover dynamics, and other Earth system processes over extended periods, helping to understand trends and patterns in a consistent manner.
Time Efficiency and Accessibility
ARD reduces the time and effort required for data preprocessing and preparation. By providing analysis-ready data, it eliminates the need for users to perform repetitive and resource-intensive preprocessing steps, such as radiometric and geometric corrections.
“ARD is a function of context”
ARD means something different to different users; in other words, it depends on the use-case⁴.
For example, a researcher who wants to apply their novel method for atmospheric correction, certainly wouldn’t access a L2-product that has already been corrected.
On the other hand, if the task is detecting vegetation change, then users would need a product they could use out-of-the-box (geometrically/radiometrically/atmospherically corrected, provided with clouds masks, etc).
Landsat ARD — The first ARD product
In 2017 the United States Geological Survey (USGS) Earth Resources Observation and Science (EROS) Center made available Landsat ARD for the conterminous United States (CONUS), Alaska, and Hawaii.²
They set the foundations of what ARD should look like. This was a massive undertaking as USGS processed decades of Landsat data.
These are the points on what ARD products should be, according to [2]:
tiled
georegistered
top of atmosphere (TOA) and atmospherically corrected
defined in a common equal area projection
accompanied by spatially explicit quality assessment information (QA bands)
have non-target features (clouds) and poor quality observations flagged
accompanied by appropriate metadata to enable further processing while retaining traceability of data provenance
geometrically and radiometrically consistent
processed in a community endorsed manner
The USGS had recognized early on that different users have different expectations from an ARD product; some might require atmospherically corrected data, while others may wish to apply their own atmospheric correction methods. So they provided both!
Also, they provide appropriate metadata, such as view and azimuth angles, so that users have all the parameters they need to further process the data (e.g. when deriving a Nadir BRDF-Adjusted Reflectance (NBAR) product).
I encourage you to read the paper [2]. The authors describe the steps they took and the challenges they faced in order to release Landsat ARD Collection 1. (the archive has been reprocessed to Collection 2 in 2020).
A prime feature of the Landsat ARD is its georegistration consistency and the main motivation for re-processing Collection 1 was to improve the Landsat absolute geolocation accuracy even further, using the Sentinel-2 Global Reference Image (GRI).
This improved the interoperability of the global Landsat archive spatially and temporally.
Achieving high geolocation enables the reliable information extraction from time series (that is, the same pixel corresponds to the same area through time and is not shifted due to misalignment issues).
This feature makes Landsat Collection 2 an “interoperable” product; set of data, originating from different sensors (Landsat 1–9), that can be stacked into a spatiotemproal data cube.
However, Landsat Collection 2 is not a harmonized product (across different Landsat missions).
Harmonized Landsat and Sentinel-2 (HLS)
“The Harmonized Landsat and Sentinel-2 (HLS) project is a NASA initiative aiming to produce a Virtual Constellation (VC) of surface reflectance (SR) data acquired by the Operational Land Imager (OLI) and MultiSpectral Instrument (MSI) aboard Landsat 8 and Sentinel-2 remote sensing satellites, respectively.”⁵
The HLS product merges observations of the land surface from both sensors into a single comprehensive dataset, leveraging their similar spectral, spatial, and angular characteristics, as well as their sun-synchronous orbits.
As the HLS team stated, by “harmonized,” they mean that the products are:
gridded to a common pixel resolution, map projection, and spatial extent
atmospherically corrected and cloud-masked to surface reflectance using a common radiative transfer algorithm,
normalised to a common nadir view geometry through Bi-directional Reflectance Distribution Function (BRDF) estimation, and
adjusted to represent the response from common spectral bandpasses.
In essence, these harmonized products serve as the foundational “data cube,” allowing users to examine any pixel over time and treat the near-daily reflectance time series as if they originated from a single sensor; this is a main characteristic of harmonisation.
(More on the differences between interoperability and harmonisation later on)
The potential of the HLS data set lies in its ability to support various applications requiring high temporal and spatial resolutions, with crop monitoring being one of the main drivers.
ARD Workshops
ARD has been such a ̶p̶a̶i̶n̶ challenge, that people organise workshops about it; Namely, the ARD Satellite Data Interoperability Workshop. Also, ARD-related sessions and talks have been taking place in the Joint Agency Commercial Imagery Evaluation (JACIE) and the Very High-resolution Radar & Optical Data Assessment (VH-RODA) Workshops.
Going through the presentations of this year’s workshops (and other recent presentations), I have highlighted some points I have found interesting:
Simon Oliver made the case for an “ARD Measurement Quality Goal” through an intercomparison study of data sources for the Digital Earth Australia program. The comparative cases presented:
Spectral difference between Landsat 8 OLI and Sentinel-2 MSI
Intercomparison between USGS Landsat 8 SR product with ESA Sentinel-2 SR
Inter-comparison between Geoscience Australia (GA) Landsat 8 and Sentinel-2 SR products
Compare multi-sensor time-series of spectral indices NDVI, NDWI, NBR generated from multiple ARD sources over typical Australian sites
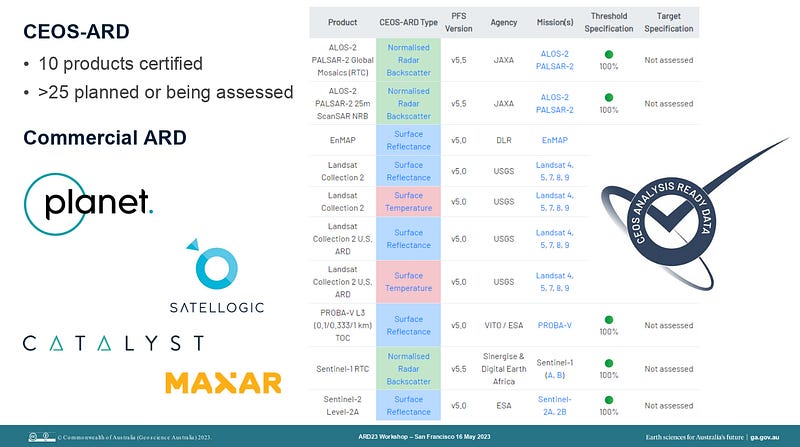
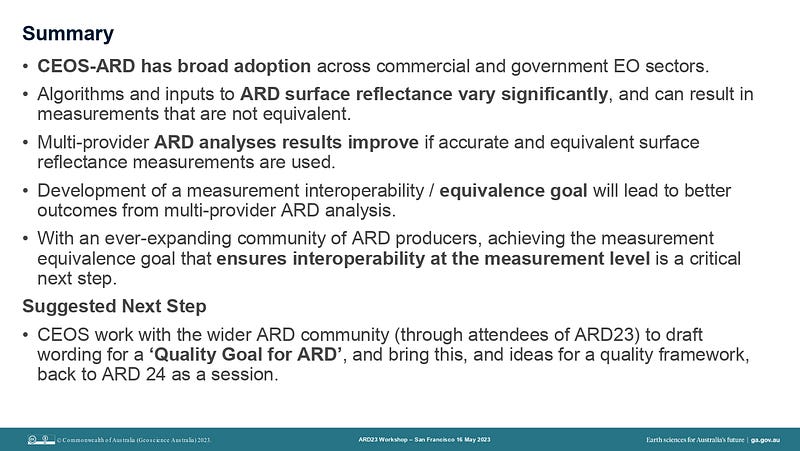
2. “ARD is a function of context”
Scott Arko from Descartes Labs had this line in their presentation, which I have used multiple times in this post; I believe it’s a core concept in ARD.
As already mentioned, ARD depends on the use case.
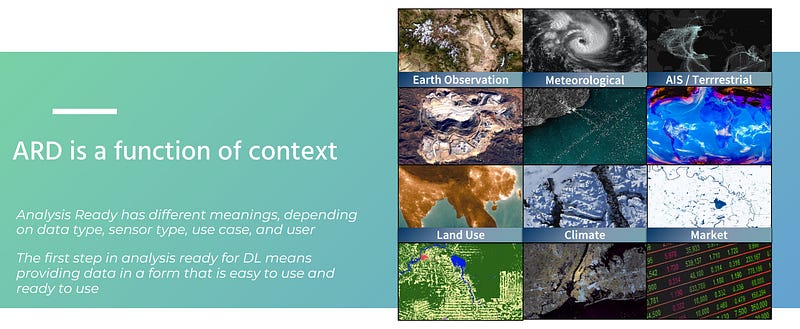
3. Sen2Like: a processor for harmonising and fusing optical EO data imagery | Enrico Giuseppe Cadau, Serco for ESA, et al. | 2023 ARD Satellite Data Interoperability Workshop
“The Sen2Like demonstration processor has been developed by ESA in the framework of the EU Copernicus programme.
The main goal of Sen2Like is to generate Sentinel-2 like harmonized/fused surface reflectances with higher periodicity by integrating additional compatible optical mission sensors.
It is a contribution to on going worldwide initiatives (*NASA-HLS, FORCE⁶, CESBIO) undertook to facilitate higher level processing starting from harmonized data.”⁷
Sen2Like performs the following steps, depening on the input product type:
Image stitching of the different tiles
Geometric corrections including the co-registration to a reference image
Inter-calibration (for S2 L1C)
Atmospheric corrections
Transformation to Nadir Bidirectional Reflectance Distribution Function (BRDF) Adjusted Reflectance (NBAR)
Application of Spectral Band Adjustment Factor (SBAF) (for LS8/9)
Production of LS8/LS9 high resolution 10 m pixel spacing data (data fusion)
Sen2Like generates the two following ARD product types:
Level-2H: harmonized Sentinel-2 and Landsat 8/9 products (native spatial resolutions kept)
Level-2F: fused Landsat 8/9 to Sentinel-2 products (S2 spatial resolution for Landsat data)
Furthermore, the team has performed studies on the integration of hyperspectral data (DESIS, PRISMA) into Sen2Like! They have proven that the Sen2Like framework is able to process the products of any other multispectral or hyperspectral optical data at the L2A level.⁸
4. “Sometimes ARD is challenging to integrate and not a good fit”
Floodbase has a really good argument for not using ARD; “Disaster response requires data ASAP”.
This isn’t exactly ARD-related, but rather using Data-As-Ready-As-It-Gets.
However, one of Floodbase’s concerns is the ability to trace errors and know whether a product is pulled and replaced! To their use-case, traceability on this level matters!
5. Greg Stensaas, USGS EROS Cal/Val Center of Excellence (ECCOE) on “The Importance of Calibration and Validation in a Changing World”
Q. “How do you get thousands of Earth-observation systems that were built at different times by different people for diverse purposes and that use dissimilar data formats and communications techniques to operate together smoothly and from a coherent system?”
A. “The only real answer is harmonisation”!!!
We live in exciting times for Earth Observation and the Satellite Industry in general. Just by looking at the chart of satellites launched, it is evident that the satellite industry is booming; a trend that is expected to continue its forward trajectory and keep driving an expanding space economy.
Landsat-8/9 and Sentinel-2A/B provide freely available data at an unprecedented scale and combining them to build and publish a harmonized product is a monumental challenge. And these are just four satellites.
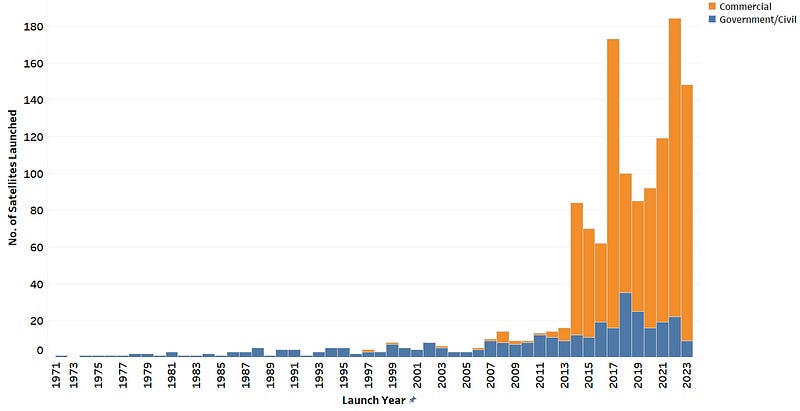
Data interoperability with Planetscope
Planet launched their first satellite in 2013. Now, they have approximately 200 satellites in orbit (~180 Doves and 21 SkySats), capturing over 25 TB of imagery daily!
Having that large a fleet of satellites, it’s not a surprise that they have a heavy presence in workshops like the ARD Satellite Data Interoperability Workshop and JACIE.
Many of their talks over the last few years have been focused on their CalVal processes and the challenges they face, as they have put huge efforts into improving their delivered imagery.
“Interoperability IS usability”
Planet’s fleet of Dove satellites offers global daily coverage and people have been trying to make the most out of this catalogue.
Many studies have used Planetscope data to fill the gaps between Landsat-8/Sentinel-2 observations and create dense time series, compare crop yield models that utilise different source data (Landsat-8/Sentinel-2/PlaneScope) or even sharpen Sentinel-2 images using 3m Planetscope data¹⁰.
However, most studies have either used a limited number of Planetscope images, or have blindly used Planet’s Level 3B analytic product. Studies such as [11], that used a combination of Planetscope/Sentinel-2/Landsat-8 imagery for crop yield estimation, had even identified the differences in surface reflectance and indices values among the different sensors, without going a step further to provide an explanation for this inconsistency. In [12], the authors studied crop yield using Planetscope data, but it seems incomplete. They developed a method based on Planetscope data but the comparison of their Random Forest model using Sentinel-2 data is “unfair”. Moreover, Random Forest models aren’t the best method to compare different data sources and to decide whether “Planetscope data are better for this task than Sentinel-2”.
It is evident that in order to derive higher level products, develop applications and make fair comparisons of among methodologies, researchers need an interoperable and harmonized dataset; a harmonized product of Planetscope data based on a “gold reference” (Sentinel-2 / Landsat-8/9).
Towards this goal, Planet has been focused on their Planet Fusion ARD product, which they have presented in recent workshops.
When operating that large a fleet of satellites there are two sets of challenges:
Cross-calibrate to a reference sensor (e.g. Sentinel-2)
Ensure intra-fleet consistency ; hundreds of cubesats with potentially different relative spectral responses
During JACIE 2023, Alan Collison et al., presented Planet’s Calibration Methodology¹³.
Planet’s calibrations are based on a collection of near simultaneous crossovers between each SuperDove and Sentinel-2 (as a reference satellite). See the Figure below.
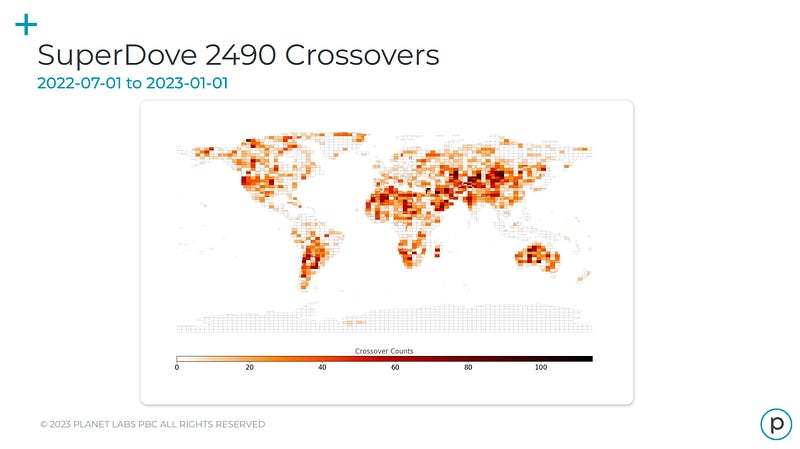
And in order to validate calibration corrections and improve consistency within the SuperDove fleet, they analyze SuperDove crossovers.
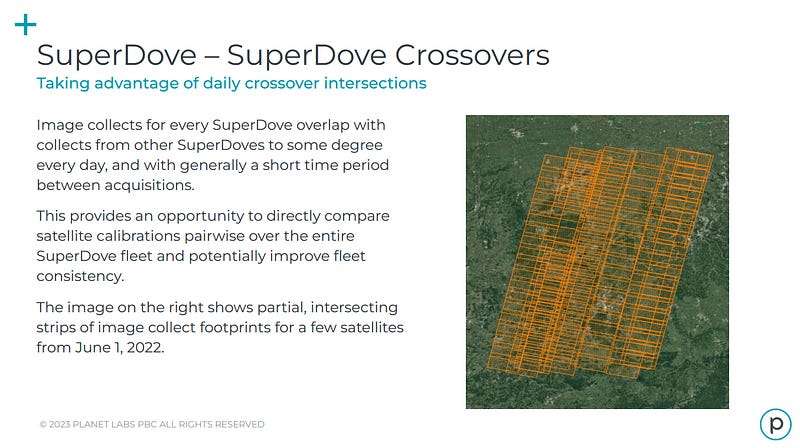
The Planet Fusion ARD Product
“The Planet Fusion processing translates original PS Top Of Atmosphere (TOA) reflectance inputs into Surface Reflectances (SR) consistent with Landsat 8 and Sentinel-2 clear-sky observations.”¹⁴
Over the last few years, folk at Planet have been trying to make use of the latest studies and frameworks (CESTEM, FORCE⁶) and combine several input data sources (MODIS/VIIRS/S2/L8) to derive a harmonized product that is consistent against other Surface Reflectance products (e.g., NASA HLS, ESA sen2cor, sen2like, USGS LaSrc).
Planet Fusion performs these top level steps:
Radiometric harmonisation
Geometric harmonisation
Cloud/Shadow masking (QA product)
Check out the processing chain of the Fusion product in the slides below.
We can grasp the scale of this challenge in the following slide as a result of the variability and uncertainty in the tasks involved¹⁵.
Even subtasks in the processing chain, like estimating Aerosol Optical Depth (AOD) and robustly detecting clouds and cloud shadows are non-trivial. Errors made in the processing chain propagate to the end product affecting its quality.
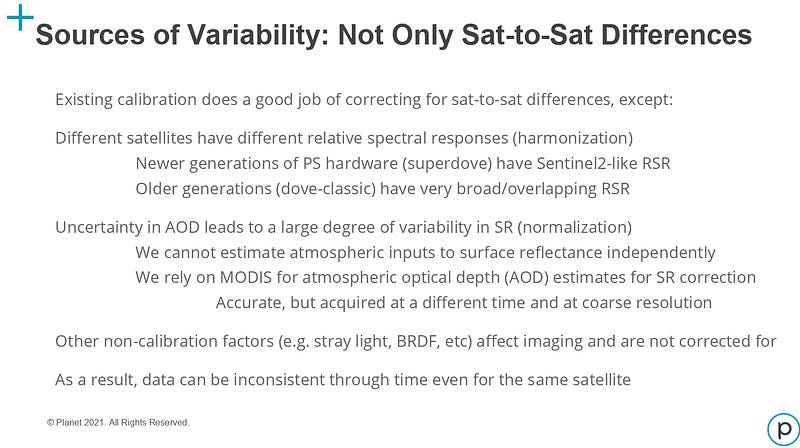
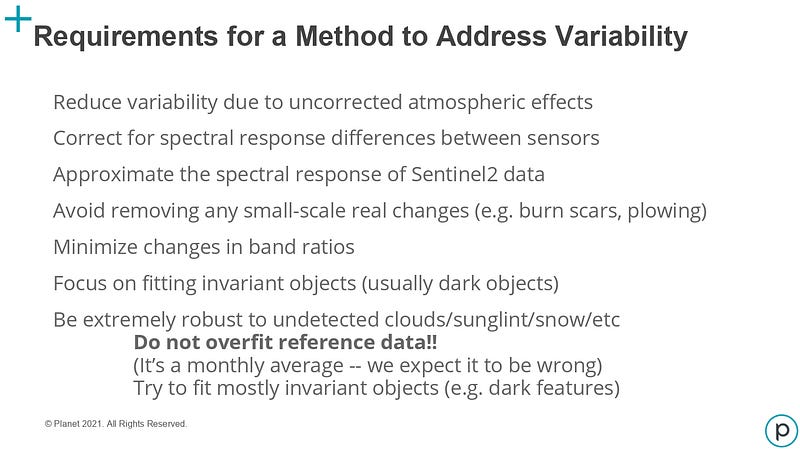
I have dedicated a large part of this section to discussing Planetscope data and the efforts to achieve interoperability with other surface reflectance products such as the HLS.
I felt this was essential to further highlight the significance of harmonized data in Earth observation. Planetscope’s extensive fleet of satellites, capturing global daily coverage and producing vast amounts of imagery, has tremendous potential for driving research and applications in various fields, including agriculture, environmental monitoring, and land cover analysis.
By exploring the challenges faced by Planet in achieving interoperability with other surface reflectance products, we gain insights into the complexities of harmonizing data from diverse sensors and sources. This in-depth examination sheds light on the crucial role of harmonisation in making data from multiple sensors compatible and standardised for further analysis and application development.
Section 2
Looking Ahead: The Water’s Edge of the USGS National Land Imaging Program
Building the Global Commons of Earth Observation Data
Last year, the Landsat Advisory Group conducted a study exploring earth observation products, including data, algorithms, and workflows.³ Their findings emphasized the significance of ARD products in achieving a harmonized and standardised collection of earth information. In its findings, they argue that the explosion in the accessibility of earth observation data combined with the rise of ARD Harmonisation products, open the possibility to form, what they refer to as, the “Global Land Imaging ARD commons”.
The aim of this concept is to combine data coming from disaggregated sensors (thermal, multispectral, hyperspectral or RADAR sensors) that are flown on government or commercial satellites, into a coherent, standardised and consistent record of Earth’s change.
As described in the paper, this platform of “commons” is not just refering to data commons but also a commons of standards that facilitate multi-sensor harmonisation for end-users.
To achieve harmonisation, it is essential to establish standards that guide data providers in cleaning and aligning their data to the ‘gold’ standard. Ideally, there should also be standards set at lower quality levels to accommodate sensors of differing quality than Landsat and Sentinel-2. This ensures that diverse sensors can be incorporated into the global commons with clear communication of their quality levels.
An important aspect of these standards is their sensor-agnostic nature, working at a higher level to allow any new sensor meeting appropriate calibration targets to seamlessly integrate into the global commons of analysis-ready earth observation data. This inclusivity ensures that the initiative remains dynamic, accommodating a wide range of sensors from different sources.
The study identified NASA’s calibration resources (such as the Goddard AERONET) as a key component of making Landsat the “gold” standard and envisions a “global sensor calibration commons” that enables interoperable data collections.
Interoperability and harmonisation in this ARD platform of “commons”
Before reading this paper I had overlooked the nuanced differences between interoperability and harmonisation.
As the paper mentions, two ARD products can be interoperable and not harmonized!
For example, Planet’s SuperDoves capture, among other spectral bands, the “Green I” and “Yellow” bands, both of which have no Sentinel-2 equivalents. Instead of excluding a piece of data from the Global Land Imaging record, the approach of this proposed platform of commons is to take advantage of the diverse spectral characteristics of each sensor, as “any measurement in the passive optical EM spectrum is relevant to earth’s changes over time”.
According to the study, this spectral and spatial diversity is what increases the overall value of the product, as each piece of information contributes to the same mission:
Build a coherent, consistent record of Earth’s change.
“Similar diversities are present when considering Landsat/Sentinel-2. Landsat OLI and Sentinel MSI sensors have unique spectral bands that add to the diversity of any product derived from the harmonized and/or fused sources that include Landsat.”
Moreover, when considering this dynamic between interoperability & harmonisation, the study proposes the scheme HLS + X, “where X is virtually any other sensor that shares the same mission, easily incorporating diverse spectral characteristics into a harmonized output.”
Creating and implementing this platform of commons presents significant challenges. However, the HLS+X interoperable data cube holds immense potential, providing users with unparalleled insights into Earth’s changes like never before.
Further Discussion on Interoperability, Harmonisation, Fusion and ARD for ML
As I’ve already mentioned, while reading the material related to the topic and after completing a draft of Section 2 I started thinking about Interoperability, Harmonisation and Fusion and the subtle differences among them. In some cases these terms are used quite loosely in literature, leaving room for interpretation.
Therefore, clarifying these concepts is crucial in enhancing our comprehension of the distinctions between various data products and the essential processes involved.
Interoperability, Harmonisation, Fusion
Interoperability
Given the information presented in the sources of this post, an interoperable product relies on geometrical consistency; that is, common geometric corrections are applied to all data sources that comprise the overall data product.
The main characteristic of an interoperable product is the ability to examine any pixel in time without experiencing missalignment issues.
Harmonisation
As mentioned earlier, a product can be interoperable without being harmonized (if geometrically consistent, a combination of Sentinel-1 and Sentinel-2 images can form an interoperable data cube!).
So, to create a harmonized product, interoperability is a prerequisite.
Here, the main characteristic of a harmonized product (comprised of at least two different sensors) is being able to treat the time series data as if they originated from a single sensor.
To achieve this harmonisation consistency, there are several steps needed, such as making spectral band adjustments and applying atmospheric corrections using common radiative transfer models and methods.
For obvious reasons, harmonisation assumes a certain level of compatibility among the different sensors combined. For example, a Sentinel-1 (SAR) image can not be harmonized to a Sentinel-2 (Optical) image. And even two images coming from two different optical sensors require compatibility (at least spectrally). For example, the yellow band captured by Planet’s Doves has no Sentinel-2 spectral equivalent to use as reference and therefore cannot be harmonized. (Some have tried to derive synthetic bands when not provided “natively” by the sensor; in [16] the authors derived synthetic Planetscope red-edge and SWIR bands using linear regression of the Planetscope visible and NIR bands with the Sentinel-2 red-edge and SWIR bands).
Fusion
In this post, there are two references to “fused/fusion” products; Sen2Like Fused Surface Reflectance Products (Level 2F), where Landsat-8/9 images are upsampled to the 10/20m native resolution of Sentinel-2 and Planet Fusion.
However, in literature, the word fusion often relates to a method that combines two different data sources; this might be a method that combines different modalities, such as SAR and optical, or even input images with different spatial resolution and/or spectral bands.
Harmonized data in the context of Machine Learning
Harmonized data is of paramount importance when it comes to training ML models.
Inconsistent data from different sensors with varying spectral characteristics and spatial resolutions can introduce noise and confounding factors that hinder the model’s ability to identify reliable patterns and make accurate predictions. On the other hand, harmonized data ensures that the input features are standardised and aligned across different sensors, making it easier for ML models to grasp underlying patterns, detect changes over time, and achieve better generalisation.
“ARD is a function of context”, and for the ML folk the context is ML-related.
Again, individual use-cases for different ML-based tasks might have different requirements. For example, for the task of cloud detection, users would need images with… clouds! And for agricultural application (crop yield estimation), the data cube would be comprised of cloud-free images.
This highlights the significance of anticipating diverse use-cases and offering clear and concise “usage instructions” tailored to various user types. Building upon the earlier examples of cloud detection and crop yield estimation, it is expected that both tasks would utilise training data from a harmonized ARD product. However, the inclusion of appropriate metadata would enable users estimating crop yield to effectively remove cloudy images from the original data, ensuring the analysis is based on cloud-free and reliable imagery.
Conclusions
Section 1 — Defining ARD and Its Importance
In Section 1, we have delved into the various definitions of “traditional” Analysis Ready Data (ARD). ARD is crucial for achieving interoperability, enabling users to analyse data from different sensors.
The release of Landsat ARD and the subsequent HLS laid the foundations of ARD in Earth Observation.
Now, the efforts have been focused into integrating additional compatible optical mission sensors to the “gold” standards of Sentinel-2 and Landsat-8/9. Sen2Like and Planet Fusion have been stepping towards that direction, facing several challenges along the way.
Section 2 — Global Land Imaging ARD commons
In Section 2, we have highlighted some of the topics of the Landsat Advisory Group’s study that gave us a glimpse into its vision of ARD and what implementing this “Global Land Imaging ARD commons” entails.
It’s a set of commons:
a commons of EO data,
a commons of standards and
a commons of global sensor calibration
where “any new sensor can add to the overall measurement of what is happening on earth”.
Having disaggregated sensors contribute to this platform of commons and the introduction of HLS+X expands the concept of ARD and makes it diverge from our original understanding.
Instead of having a coherent, consistent, harmonized datacube “ready-to-be-explored”, in this new HLS+X datacube we have every available piece of data for an area in time.
This, to me, is a major shift and it makes a few related concepts such as “interoperability, harmonisation, fusion” more entangled.
Harmonizing planet data
The pursuit of harmonisation between Planetscope data and other surface reflectance products aligns with the vision of creating a harmonized Global Land Imaging Analysis Ready Data (ARD) commons. Such an initiative holds the promise of fostering a dynamic and collaborative data ecosystem, where harmonized data sets can be seamlessly integrated and utilised by researchers and stakeholders across the globe. Ultimately, by understanding the efforts made towards interoperability and harmonisation, we gain a clearer understanding of how standardised data can unlock the full potential of Earth Observation and lead to groundbreaking advancements in understanding our planet’s changes and challenges.
Going Beyond Analysis Ready Data
Both the “traditional” and “expanded” versions of ARD serve as crucial foundations for deriving higher-level products in Earth Observation.
The concept of ARD has proven its significance in achieving data interoperability, consistent time-series analysis, and time efficiency for various user applications.
However, going beyond ARD opens up new possibilities for the Earth Observation community. By integrating additional compatible optical mission sensors, as demonstrated by Sen2Like and Planet Fusion, the Global Land Imaging ARD commons envisions a dynamic and collaborative data ecosystem. This platform of “commons” not only includes data but also encompasses standards and global sensor calibration, enabling diverse sensors to contribute to a coherent and consistent record of Earth’s changes.
Looking ahead, embracing the idea of a data cube that accommodates various sensors and spectral characteristics will undoubtedly push the boundaries of Earth Observation and lead to groundbreaking advancements in understanding our planet’s complexities and addressing pressing global challenges.
Afterthoughts
Redefining ARD
Throughout this exploration of ARD in Section 1 and the expanded version in Section 2, it becomes evident that the definitions and scope of ARD have evolved significantly.
As we encounter different interpretations and applications of ARD, a crucial question arises:
Should both the “traditional” and “expanded” versions of ARD be labeled under the same term?
The divergence in definitions and objectives calls for a re-evaluation of the term “ARD” itself. While the original concept served as a foundation for ready-to-use data, the expanded version embraces a Global Land Imaging ARD commons with a focus on data interoperability and inclusivity of diverse sensors. Perhaps it is time to consider redefining ARD to encompass its evolving nature and to differentiate between these distinct but complementary concepts.
A Recipe-Based Approach for ARD?
While researching the topic and writing this article, I couldn’t help but consider whether ARD could be effectively implemented through a recipe-based approach, utilising configuration files with distinct models and parameters for various stages of data processing? This recipe-concept envisions a modular workflow where raw data undergoes a series of internal products and transformations, eventually leading to the creation of the final ARD product; custom-made to the specific use-case with full traceability. Similar to following a recipe while cooking, each step would be guided by predefined configurations, making use of open-source methods and tools, akin to some of the configurable processes employed by FORCE and Sen2Like.
By adopting such a recipe-based workflow, users could experiment with different combinations of algorithms and parameters, providing greater flexibility and customization in generating ARD products that align with specific use-cases.
Additionally, embracing algorithm sharing and social coding principles could foster collaborative development within the Earth Observation community, encouraging experts to openly contribute to the improvement of algorithms and data processing techniques.
However, balancing the intellectual property (IP) value of proprietary algorithms with the openness of open-source code would require careful consideration to ensure transparency, reproducibility, and accessibility of the ARD recipes and associated tools.
Feedback and corrections
While I’ve tried to accurately represent the insights and ideas from the presentations and sources discussed in this article, the complex nature of the subject may have led to unintended misinterpretations or omissions.
To both the contributors of the materials referenced and readers, I invite you to share any corrections, clarifications, or additional context that could enhance the accuracy and depth of this article.
Acknowledgments
Many thanks to Gary Crowley and Katerina Bakousi for their invaluable feedback, insightful comments and engaging discussions that significantly contributed to the refinement of this article.
Sources
[1] Sentinel-1 ARD Normalised Radar Backscatter (NRB) Product [link]
[2] Dwyer, John L., et al. “Analysis ready data: enabling analysis of the Landsat archive.” Remote Sensing 10.9 (2018): 1363.
[3] Looking Ahead: The Water’s Edge of the USGS National Land Imaging Program — Building the Global Commons of Earth Observation Data [link]
[4] Deconstructing Analysis-Ready Data [link]
[5] Claverie, Martin, et al. “The Harmonized Landsat and Sentinel-2 surface reflectance data set.” Remote sensing of environment 219 (2018): 145–161.
[6] Framework for Operational Radiometric Correction for Environmental monitoring (FORCE) [link]
[7] https://github.com/senbox-org/sen2like
[8] Saunier, Sébastien, et al. “Sen2Like: Paving the Way towards Harmonization and Fusion of Optical Data.” Remote Sensing 14.16 (2022): 3855.
[9] Planet Fusion Monitoring — Rasmus Houborg, Planet [link]
[10] Li, Zhongbin, et al. “Sharpening the Sentinel-2 10 and 20 m Bands to Planetscope-0 3 m Resolution.” Remote Sensing 12.15 (2020): 2406.
[11] Tunca, Emre, Eyüp Selim Köksal, and Sakine Çetin Taner. “Silage maize yield estimation by using planetscope, sentinel-2A and landsat 8 OLI satellite images.” Smart Agricultural Technology 4 (2023): 100165.
[12] Nieto, Luciana, et al. “Impact of High-Cadence Earth Observation in Maize Crop Phenology Classification.” Remote Sensing 14.3 (2022): 469.
[13] Challenges for the Radiometric Interoperability of a Mixed Fleet of Medium Res, High Res and Hyperspectral Satellites — Alan Collison, Planet [link]
[14] Planet Fusion Monitoring Technical Specification, Version 1.0.0, March 2022 [link]
[15] Per-Scene Harmonization & Normalisation of Planetscope Data — Joe Kington, Planet [link]
[16] Li, Zhongbin, et al. “Sharpening the Sentinel-2 10 and 20 m Bands to Planetscope-0 3 m Resolution.” Remote Sensing 12.15 (2020): 2406.
Wow, this is very comprehensive. Thanks for doing all that reading and interpretation so I don't have to.